时间序列模型使用流程
AR, MA, ARMA, and ARIMA models are used to forecast the observation at (t+1) based on the historical data of previous time spots recorded for the same observation. However, it is necessary to make sure that the time series is stationary over the historical data of observation overtime period. If the time series is not stationary then we could apply the differencing factor on the records and see if the graph of the time series is a stationary overtime period.
AR,MA,ARMA和ARIMA模型用于基于为同一观测记录的先前时间点的历史数据来预测(t + 1)处的观测。 但是,有必要确保时间序列在观察超时时段的历史数据上保持平稳。 如果时间序列不是平稳的,那么我们可以在记录上应用差异因子,然后查看时间序列的图是否是平稳的加班周期。
ACF(自动相关功能) (ACF (Auto Correlation Function))
Auto Correlation function takes into consideration of all the past observations irrespective of its effect on the future or present time period. It calculates the correlation between the t and (t-k) time period. It includes all the lags or intervals between t and (t-k) time periods. Correlation is always calculated using the Pearson Correlation formula.
自相关功能会考虑所有过去的观察结果,无论其对未来或当前时间段的影响如何。 它计算t和(tk)时间段之间的相关性。 它包括t和(tk)时间段之间的所有延迟或间隔。 始终使用Pearson Correlation公式计算相关性。
PACF(部分相关函数) (PACF(Partial Correlation Function))
The PACF determines the partial correlation between time period t and t-k. It doesn’t take into consideration all the time lags between t and t-k. For e.g. let's assume that today's stock price may be dependent on 3 days prior stock price but it might not take into consideration yesterday's stock price closure. Hence we consider only the time lags having a direct impact on future time period by neglecting the insignificant time lags in between the two-time slots t and t-k.
PACF确定时间段t和tk之间的偏相关。 它没有考虑到t和tk之间的所有时滞。 例如,假设今天的股价可能取决于之前三天的股价,但可能没有考虑到昨天的股价收盘。 因此,通过忽略两个时隙t和tk之间的无关紧要的时滞,我们认为仅时滞对未来时间段有直接影响。
如何区分何时使用ACF和PACF? (How to differentiate when to use ACF and PACF?)
Let's take an example of sweets sale and income generated in a village over a year. Under the assumption that every 2 months there is a festival in the village, we take out the historical data of sweets sale and income generated for 12 months. If we plot the time as month then we can observe that when it comes to calculating the sweets sale we are interested in only alternate months as the sale of sweets increases every two months. But if we are to consider the income generated next month then we have to take into consideration all the 12 months of last year.
让我们以一个村庄一年来的糖果销售和收入为例。 假设村里每2个月有一个节日,我们就拿出12个月的糖果销售和收入历史数据。 如果我们将时间绘制成月,那么我们可以观察到,在计算糖果销售时,我们仅对交替的月份感兴趣,因为糖果的销售每两个月增加一次。 但是,如果我们要考虑下个月产生的收入,那么我们就必须考虑去年的所有12个月。
So in the above situation, we will use ACF to find out the income generated in the future but we will be using PACF to find out the sweets sold in the next month.
因此,在上述情况下,我们将使用ACF来查找将来产生的收入,但是我们将使用PACF来查找下个月出售的糖果。
AR(自回归)模型 (AR (Auto-Regressive) Model)
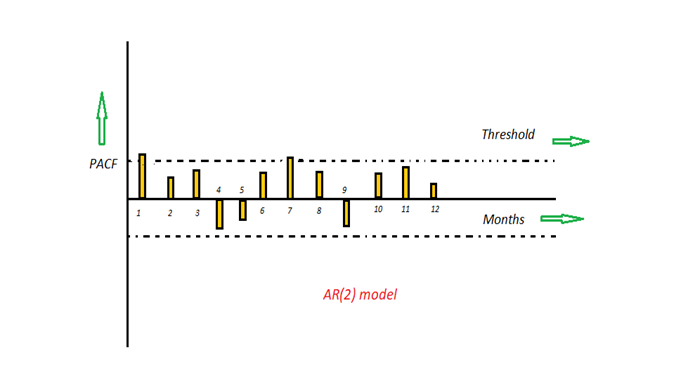
The time period at t is impacted by the observation at various slots t-1, t-2, t-3, ….., t-k. The impact of previous time spots is decided by the coefficient factor at that particular period of time. The price of a share of any particular company X may depend on all the previous share prices in the time series. This kind of model calculates the regression of past time series and calculates the present or future values in the series in know as Auto Regression (AR) model.
t的时间段受在t-1,t-2,t-3,…..tk不同时隙的观察影响。 先前时间点的影响由该特定时间段的系数决定。 任何特定公司X的股票价格可能取决于时间序列中所有先前的股票价格。 这种模型计算过去时间序列的回归,并以已知的自动回归(AR)模型计算序列中的当前值或将来值。
Yt = β₁* y-₁ + β₂* yₜ-₂ + β₃ * yₜ-₃ + ………… + βₖ * yₜ-ₖ
Yt =β₁*y-₁+β2 *yₜ-_2+β₃*yₜ-₃+…………+βₖ*yₜ-ₖ
Consider an example of a milk distribution company that produces milk every month in the country. We want to calculate the amount of milk to be produced current month considering the milk generated in the last year. We begin by calculating the PACF values of all the 12 lags with respect to the current month. If the value of the PACF of any particular month is more than a significant value only those values will be considered for the model analysis.
考虑一个牛奶分销公司的例子,该公司每月在该国生产牛奶。 考虑到去年产生的牛奶,我们要计算当月的牛奶产量。 我们首先计算相对于当月的所有12个滞后的PACF值。 如果任何特定月份的PACF值大于显着值,则仅将这些值考虑用于模型分析。
For e.g in the above figure the values 1,2, 3 up to 12 displays the direct effect(PACF) of the milk production in the current month w.r.t the given the lag t. If we consider two significant values above the threshold then the model will be termed as AR(2).
例如,在上图中,值1,2、3到12表示给定滞后t时当月的牛奶产量的直接影响(PACF)。 如果我们认为高于阈值的两个有效值,则该模型将被称为AR(2)。
MA(移动平均值)模型 (MA (Moving Average) Model)
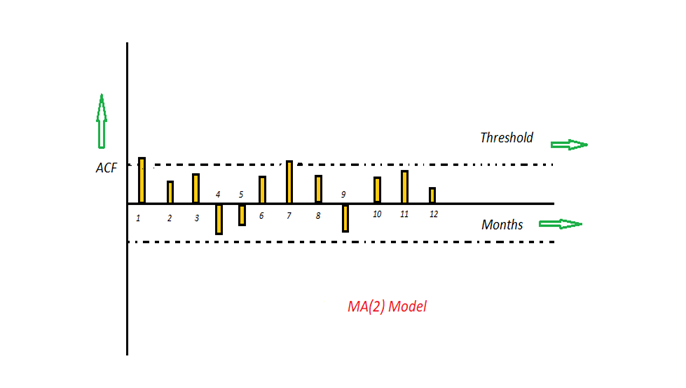
The time period at t is impacted by the unexpected external factors at various slots t-1, t-2, t-3, ….., t-k. These unexpected impacts are known as Errors or Residuals. The impact of previous time spots is decided by the coefficient factor α at that particular period of time. The price of a share of any particular company X may depend on some company merger that happened overnight or maybe the company resulted in shutdown due to bankruptcy. This kind of model calculates the residuals or errors of past time series and calculates the present or future values in the series in know as Moving Average (MA) model.
t的时间段受各种时隙t-1,t-2,t-3,…..tk中意外的外部因素的影响。 这些意外的影响称为“错误或残差”。 先前时间点的影响由该特定时间段的系数因子α决定。 任何特定公司X的股票价格可能取决于在一夜之间发生的某些公司合并,或者该公司可能因破产而倒闭。 这种模型可以计算过去时间序列的残差或误差,并以已知的移动平均(MA)模型来计算序列中的当前值或将来值。
Yt = α₁* Ɛₜ-₁ + α₂ * Ɛₜ-₂ + α₃ * Ɛₜ-₃ + ………… + αₖ * Ɛₜ-ₖ
Yt =α₁*Ɛₜ-₁+α2*Ɛₜ-²+α₃*Ɛₜ-₃+…………+αₖ*Ɛₜ-ₖ
Consider an example of Cake distribution during my birthday. Let's assume that your mom asks you to bring pastries to the party. Every year you miss judging the no of invites to the party and end upbringing more or less no of cakes as per requirement. The difference in the actual and expected results in the error. So you want to avoid the error for this year hence we apply the moving average model on the time series and calculate the no of pastries needed this year based on past collective errors. Next, calculate the ACF values of all the lags in the time series. If the value of the ACF of any particular month is more than a significant value only those values will be considered for the model analysis.
考虑一下我生日那天分发蛋糕的例子。 假设您的妈妈要您带糕点参加聚会。 每年您都会错过判断参加聚会的邀请数并最终按照要求养成或多或少的蛋糕的机会。 实际和预期的差异会导致错误。 因此,您要避免今年的误差,因此我们将移动平均模型应用于时间序列,并根据过去的集体误差计算今年所需的糕点数量。 接下来,计算时间序列中所有滞后的ACF值。 如果任何特定月份的ACF值大于显着值,则仅将这些值考虑用于模型分析。
For e.g in the above figure the values 1,2, 3 up to 12 displays the total error(ACF) of count in pastries current month w.r.t the given the lag t by considering all the in-between lags between time t and current month. If we consider two significant values above the threshold then the model will be termed as MA(2).
例如,在上图中,值1,2、3(最多12)显示了糕点在当月的总误差(ACF),其中给定的滞后t是考虑了时间t和当月之间的所有中间滞后。 如果我们认为高于阈值的两个有效值,则该模型将称为MA(2)。
ARMA(自动回归移动平均线)模型 (ARMA (Auto Regressive Moving Average) Model)
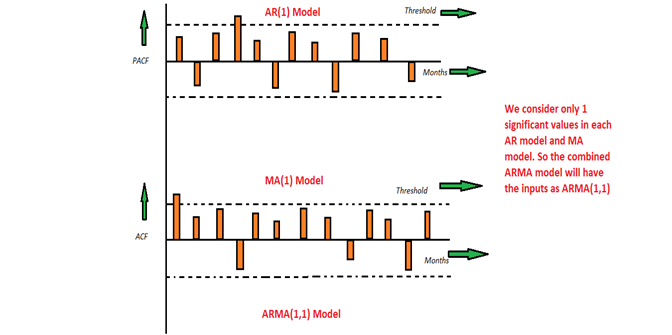
This is a model that is combined from the AR and MA models. In this model, the impact of previous lags along with the residuals is considered for forecasting the future values of the time series. Here β represents the coefficients of the AR model and α represents the coefficients of the MA model.
此模型是AR和MA模型的组合。 在此模型中,考虑了先前滞后的影响以及残差,以预测时间序列的未来值。 这里,β代表AR模型的系数,α代表MA模型的系数。
Yt = β₁* yₜ-₁ + α₁* Ɛₜ-₁ + β₂* yₜ-₂ + α₂ * Ɛₜ-₂ + β₃ * yₜ-₃ + α₃ * Ɛₜ-₃ +………… + βₖ * yₜ-ₖ + αₖ * Ɛₜ-ₖ
Yt =β₁*yₜ-₁+α₁*Ɛₜ-₁+β2 *yₜ-²+α2 *Ɛₜ-_2+β₃*yₜ-₃+α₃*Ɛₜ-₃+…………+βₖ*yₜ-ₖ+αₖ *Ɛₜ-ₖ
Consider the above graphs where the MA and AR values are plotted with their respective significant values. Let's assume that we consider only 1 significant value from the AR model and likewise 1 significant value from the MA model. So the ARMA model will be obtained from the combined values of the other two models will be of the order of ARMA(1,1).
考虑上面的图,其中MA和AR值分别以其有效值绘制。 假设我们只考虑AR模型的1个有效值,同样考虑MA模型的1个有效值。 因此,将从其他两个模型的组合值获得ARMA模型,其数量级将为ARMA(1,1)。
ARIMA(自回归综合移动平均线)模型 (ARIMA (Auto-Regressive Integrated Moving Average) Model)
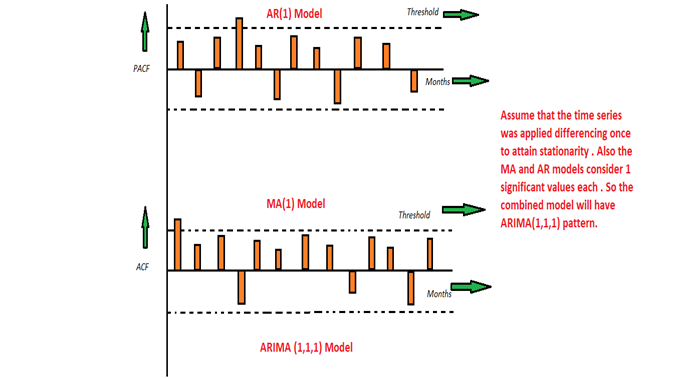
We know that in order to apply the various models we must in the beginning convert the series into Stationary Time Series. In order to achieve the same, we apply the differencing or Integrated method where we subtract the t-1 value from t values of time series. After applying the first differencing if we are still unable to get the Stationary time series then we again apply the second-order differencing.
我们知道,为了应用各种模型,我们必须在开始时将序列转换为平稳时间序列。 为了达到相同的目的,我们应用了微分或积分方法,其中从时间序列的t值中减去t-1值。 在应用一次微分之后,如果我们仍然无法获得固定时间序列,那么我们将再次应用二次微分。
The ARIMA model is quite similar to the ARMA model other than the fact that it includes one more factor known as Integrated( I ) i.e. differencing which stands for I in the ARIMA model. So in short ARIMA model is a combination of a number of differences already applied on the model in order to make it stationary, the number of previous lags along with residuals errors in order to forecast future values.
ARIMA模型与ARMA模型非常相似,只不过它包含一个称为集成(I)的因子,即在ARIMA模型中代表I的差分。 因此,简而言之,ARIMA模型是为了使模型变得平稳而已应用到模型上的多个差异的组合,其中先前的滞后次数与残差误差一起用于预测未来值。
Consider the above graphs where the MA and AR values are plotted with their respective significant values. Let's assume that we consider only 1 significant value from the AR model and likewise 1 significant value from the MA model. Also, the graph was initially non-stationary and we had to perform differencing operation once in order to convert into a stationary set. Hence the ARIMA model which will be obtained from the combined values of the other two models along with the Integral operator can be displayed as ARIMA(1,1,1).
考虑上面的图,其中MA和AR值分别以其有效值绘制。 假设我们只考虑AR模型的1个有效值,同样考虑MA模型的1个有效值。 而且,该图最初是非平稳的,我们必须执行一次微分运算才能转换为平稳集。 因此,将从其他两个模型的组合值与积分算子一起获得的ARIMA模型可以显示为ARIMA(1,1,1)。
结论: (Conclusion :)
All these models give us an insight or at least close enough prediction about any particular time series. Also, it depends on the users that which model perfectly suffices their needs. If the chances of error rate are less in any one model compared to other models then it's preferred that we choose the one which gives us the closest estimation.
所有这些模型为我们提供了关于任何特定时间序列的见识或至少足够接近的预测。 同样,取决于用户的是哪种型号完全可以满足他们的需求。 如果在任何一个模型中错误率的机会都比其他模型少,那么我们最好选择一个给出最接近估计值的模型。
Hope this article helps you to understand things better !!
希望本文能帮助您更好地理解!!
翻译自: https://towardsdatascience.com/time-series-models-d9266f8ac7b0
时间序列模型使用流程